The Spread of "China Virus" on Twitter
BERT embeddings classify reactions to Trump's inflammatory language
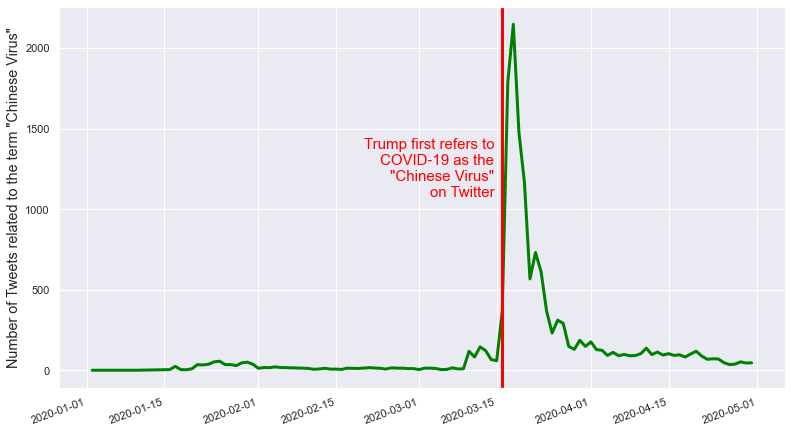
In March, as COVID-19 was undergoing its first uncontrolled spread throughout several US states, President Donald Trump began referring to the disease as the “Chinese Virus”. His tweet was widely commented and shared, and garnered over 150,000 likes. It also triggered scores of political reactions, anti-Asian discrimination, and thousands of subsequent social media posts on the topic.
In this post, we use Natural Language Processing (NLP) to analyze the discourse around the term “Chinese Virus” on Twitter. We find that the conversation was largely generated by President Trump’s use of the term, and that geographic variations in those supporting or criticizing the term largely follow partisan political lines. These results demonstrate that political leaders have a very strong influence on how their followers use language, and point to a deeply polarized country.
One Tweet can go a long way
Donald Trump first used “Chinese Virus” on Twitter on March 16th, claiming that COVID-19 “comes from China” when pressed on the issue. A widespread debate ensued, with many health experts cautioning against the use of the term because of the stigma it might cause and with Democratic politicians decrying it as racist. In the subsequent months, however, expressions such as “Chinese Virus”, “Wuhan Virus”, and even “Kung Flu” have become commonplace in Trump Administration communications, among right-wing commentators, and on social media. Anti-Asian harassment linked to the COVID-19 pandemic has become a global issue, with most attacks placing blame on Asians for causing the virus or spreading it to others. In the US, the Stop AAPI Hate organization recorded over 2,500 incidents of anti-Asian violence from March 19th through August 5th. Inflammatory language that blames Chinese people for the pandemic undoubtedly fueled this violence.
Cuomo wants "all states to be treated the same." But all states aren’t the same. Some are being hit hard by the Chinese Virus, some are being hit practically not at all. New York is a very big "hotspot", West Virginia has, thus far, zero cases. Andrew, keep politics out of it....
— Donald J. Trump (@realDonaldTrump) March 17, 2020
For the purpose of this project, we collect all the tweets related to the term “Chinese Virus” from January 1st, 2020 to April 30th, 2020. Overall, 14,984 posts are included in our analysis. Initial time-series plots show that while the term was already in use within small circles before Donald Trump’s tweet, its use went through the roof in the days following the president’s endorsement. Since we restrict our analysis to geotagged content, we are able to pinpoint where users were located when they reacted to the president’s tweet. The county-level map below shows that reaction and subsequent use of the term “Chinese Virus” took place across the entire country.
As could be expected, much of the social media activity was concentrated in dense urban areas such as Miami, Los Angeles, Houston, and New York. In fact, at state level, prevalence of the topic is mostly proportional to population. Largely, the term “Chinese Virus” was on the mind of social media users everywhere, and number of related posts is surprisingly independent of state-level political leanings and socioeconomic characteristics. One notable exception is the District of Columbia—where the topic was remarkably salient within a small population, likely due to the concentration of news outlets and political users.
Opinions expressed, however, were radically polarized. Some users rushed to denounce the term “Chinese Virus” as racist. For others, its unapologetic use by national elites normalized the term, which became an acceptable synonym to “COVID-19”. Finally, in specific far-right circles, this tweet fueled dozens of conspiracies on the inception and spread of the virus.
NLP analysis highlights polarized reactions
Understanding the nature of the reaction therefore requires classifying the tweets based on the point of view they express. Advanced methods of NLP can create representations of social media posts which account for their lexical field, syntax, and structure. Here, we use a state-of-the-art transformer language model called BERT to create easy-to-compare representations. Similar representations—characterizing tweets with similar expressed topics, opinions, and sentiment—are classified into clusters of semantic meaning.
Since our classification method is unsupervised, we don’t know a priori what point of view will be expressed in each cluster. We approximate the meaning of every cluster by printing the 5 tweets most central to that cluster. When the clusters are relevantly defined, these tweets have similar semantic meaning and are representative of the overall opinions and sentiment expressed in the cluster.
An initial, 3-cluster classification of the already produces relevant splits in the data. Cluster 2 is made up of mostly short, uninformative tweets using “Chinese Virus”. However, clusters 1 and 3 distinguish content denouncing the use of the term (Cluster 3) from content supporting its use (Cluster 1).
Cluster 1 (6236 tweets) is approximated by: - BREAKING ☣ NEWS Major Virus Model Updated, Projected US Deaths Drop to Zero by June 19th ✅ #CCP #COVID19 #Wuhan #ChinaVirus #MadeInChina Download The Epoch Times app to see our exclusive Coronavirus coverage and daily updates - #happeningNow US President Trump giving an update on what he says is ‘the war on the Chinese Virus’. The virus he once called the Democrat’s hoax. And later said it was under control and would disappear through a miracle. @realDonaldTrump #coronavirus #StimulusPackage2020… - Order your Herbalife products today order today and follow me on here and Instagram receive a discount today #stayhomechallenge #QuarantineLife #Covid_19 #ChineseVirus #covidindia #CoronavirusOutbreak #TomBrady #NFL #TuesdayMorning #MLBTheShow20 - #numbersdontjive #COVIDー19 #ChineseVirus Hospitalizations for COVID-19 Drop Sharply in New York Download The Epoch Times app to see our exclusive Coronavirus coverage and daily updates - @realDonaldTrump President Trump is smart to let his medical team assume full accountability regarding the trending of the #ChinaCoronavirus: White House task force provides update on the COVID-19 pandemic #Coronavirus Cluster 2 (3279 tweets) is approximated by: - It’s a Chinese Virus, right? - Call it what it is THE Chinese Virus - Bruh called it the "Chinese Virus" - Note: the Chinese Virus. - "The Chinese Virus" Cluster 3 (5469 tweets) is approximated by: - @realDonaldTrump calling it the "Chinese Virus" is the most DISRESPECTFUL, RACIST, IGNORANT thing any politician has said during this pandemic. If you still vote for this guy you are the scum of the earth. - Hey @w_terrence stop using the #ChineseVirus this what happens when you and your incompetent #POTUS says the its the #ChineseVirus people like you attack asian americans. You should be ashamed of your self - You idiot! Asians are being attacked physically & hurt because Traitor Trump calls it the Chinese Virus! He spreads racism & hatred every time he gets a chance, but youve got your nose so far up his fat butt you don't see it! Shame on you! - If @potus use of racial slur #ChineseVirus causes anybody to attack & murder any Asian @realDonaldTrump needs to be held legally responsible. It's disgusting & #unAmerican to have President use his office to incite hatred. And this is even worse than his history of racial hatred - How ignorant must one be, when the only question you have for the #POTUS is a comment asking is he a racist toward China because he referred to this as a Chinese Virus! What a world we live in now..EVERYONE IS SO HELL BENT ON FINDING SOMETHING TO BE OFFENDED OF..#Misery
As we increase the number of clusters, additional nuances start to appear. For 7 classes, the uninformative cluster remains, but the positive and negative clusters have been split into more granular categories. We can manually assign the 7 clusters to 5 categories described below.
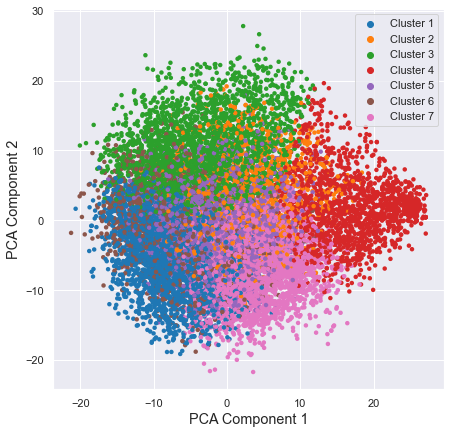
Cluster 1 (2547 tweets) is approximated by: - The beauty and serenity of America is shining through, the people of America are praying for all the sick, not only from the Chinese Virus but all illness. Dear Lord please take this cup from us and allow us to get back to living the wonderful life you have given us we pray. Amen - @MELANIATRUMP Happy Birthday the 1Lady of the USA . I wish you on side of Mr President to help him can control of the China virus make Americans strong again .God bless Americans Mr President - I voted yes b4: 2 of my grandchildren got quarantined. 2 weeks due to a distant exposure. Good news no Chinese Virus & they get sprung Friday. Happy teenage siblings who await their release. Glad I couldn't visit as they been fighting like the brother & sister teenagers they are. - @RealMattCouch and his guest made a very valid point. Why hasn't the elec, gas, cable, car companies..etc..stepped up to the plate and given the everyday average Joe a 2-3 month break from paying the bills during this Chinese Virus. - @realDonaldTrump you are right sir that this is Chinese Virus but why didn’t you cease boarder for them when they were returning to USA after Jan 19, they had returned hometown to celebrate their new year on Jan 25. We didn’t have to suffer so much Cluster 2 (1396 tweets) is approximated by: - It's NOT A Chinese Virus!! STOP IT - IT'S NOT THE "Chinese Virus." PLEASE STOP CALLING IT THAT. - It isnt the Chinese coronavirus. Just stop! - ITS NOT THE CHINA VIRUS!!! LOL - HOW ABOUT YOU START BY NOT CALLING IT THE "Chinese Virus"! Cluster 3 (2782 tweets) is approximated by: - This is one of the MANY reasons WHY #POTUS45 calling #COVID19 the #ChineseVirus is not okay and perpetuates #racism and #bias against #asian people. I’m disgusted that this is happening and others should be as well. - Me: why does he keep calling it the Chinese Virus like it doesn’t have a name 🙄 @BigEdH53 : because he’s a racist scumbag, do you really have to ask? - How ignorant must one be, when the only question you have for the #POTUS is a comment asking is he a racist toward China because he referred to this as a Chinese Virus! What a world we live in now..EVERYONE IS SO HELL BENT ON FINDING SOMETHING TO BE OFFENDED OF..#Misery - Calling this "the Chinese Virus" is very racist and disgusting - Did you really just call it the "Chinese Virus?" You’re an embarrassment and a racist POS. Cluster 4 (1724 tweets) is approximated by: - It’s a Chinese Virus, right? - Bruh called it the "Chinese Virus" - Call it what it is THE Chinese Virus - "The Chinese Virus" - "Chinese Virus" uhhh Cluster 5 (2130 tweets) is approximated by: - She does not KNOW how many cases of China virus there are ANYWHERE and if she avers otherwise, she needs to be placed into solitary confinement until further notice - @POTUS We cannot let this happen. The longer Chinese Virus runs the Country it could happen. - It’s my understanding the problem started with a good many people catching the China virus and ultimately production delays due to manpower shortages. Sounds as though leadership at this company needs a performance review. - UF will no longer approve of student or employee trips to China because of the coronavirus outbreak. Read about how this has affected students and in more detail below. - The Chinese Virus got the boomers at work terrified Cluster 6 (1975 tweets) is approximated by: - Trump = Tremendous Racist. HUGE and TREMENDOUS racist, in case it was not clear. #tremendous #chinesevirus #CancelTrump - @JoeNBC @maddow @morningmika. This is the result of Trump's racist China virus comments - « Chinese Virus »?? WTF is about Trump? @POTUS - Chinese Virus? No, it’s the Trump Virus. #TrumpVirus - Listening to Trump talk is making me sick, he really just said calling the coronavirus the "Chinese Virus" isn’t racist.... 😒 Cluster 7 (2430 tweets) is approximated by: - How #coronavirus #COVID19 all started #Wuhan #ChineseVirus @SouthPark #COVID2019 #coronapocolypse - #COVID19 #Quarantine #COVID19US #SocialDistanacing #ChinaVirus #CoronaVirusUpdate Interesting. - Look what I found on @eBay! via @eBay #coroanvirus #coronavirususa #coronavirusus #COVIDー19 #CoronaVirusUpdates #CoronavirusOutbreak #coronavirusaustralia #ChinaVirus #China - Look what I found on @eBay! via @eBay #ChinaVirus #China #coronavirususa #Coronavid19 #Coronavirusmexico #coronavirusus #Coronaviriusitalia #coroanvirus #coronavirusaustralia #coronaviruses #COVIDー19 #COVID2019 #CoronaVirusUpdates #CoronavirusOutbreak - #ChinaLiesPeopleDie #CCPVirus #ChineseCoronaVirus #ChineseBioterrorism #coronavirus #COVID19 #COVID
Clusters | Manual Interpretation | Number of Tweets |
---|---|---|
1 and 5 | Affirmative use of “Chinese Virus” | 4,677 tweets |
2 | Anger at the use of “Chinese Virus” | 1,396 tweets |
3 and 6 | Critical use of “Chinese Virus” | 4,757 tweets |
4 | Short, uninformative tweets | 1,724 tweets |
7 | Hashtags, normalized use of “Chinese Virus” | 2,430 tweets |
This aggregate analysis glosses over important temporal dynamics. The initial surge in “Chinese Virus”-related content, in immediate reaction to Donald Trump’s infamous tweet, was mostly condemning the president’s rhetoric as racist (‘Critical use of “Chinese Virus”’), or expressing surprise and disbelief (‘Short, uninformative tweets’).
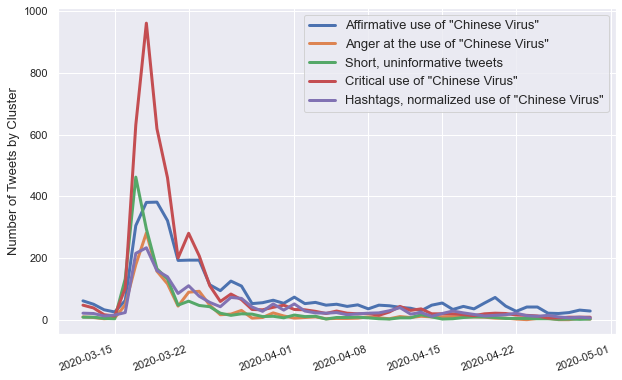
The overall number of topical tweets quickly decreased and within 2 weeks of Trump’s first use of the term, making it hard to distinguish which clusters dominate the Twittersphere subsequently. However, examining the share of clusters instead of overall numbers sheds lights on longer-term effects. While denunciation of the term as racist, and anger at its use, gradually tapers off, positive use of the term persists in certain circles. By the end of April, 60% of tweets on the topic of “Chinese Virus” use the term in an affirmative way.
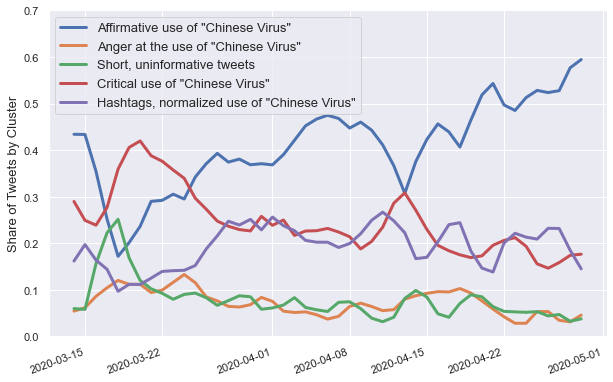
Reactions are largely location-specific
Cluster prevalence is also highly location-specific and, at the state level, the share of posts pertaining to each cluster varies importantly. We create state-level cluster scores by calculating the share of tweets in every cluster, and scaling across states. Among the states with the largest populations, New York and California exhibit higher levels of content critical of Donald Trump’s rhetoric, whereas users in Florida and Texas are more likely to support the use of the term.
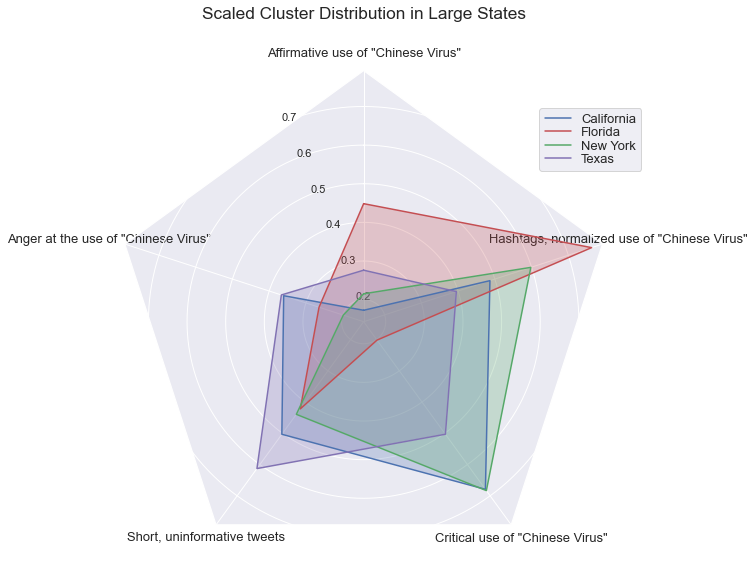
Similar analysis can be conducted in historically polarized states. In traditionally Republican states—such as Alabama, Kentucky, and Oklahoma—Trump’s rhetoric resonates with voters: the term, quasi-inexistent in social media before its use by the President of the United States, is normalized or even encouraged by users. In traditionally Democratic states, on the other hand, the reaction is strong opposition to the use of the term. The District of Columbia, Massachusetts, and Washington have among the highest scores in the country on the ‘Critical use of “Chinese Virus”’ cluster.
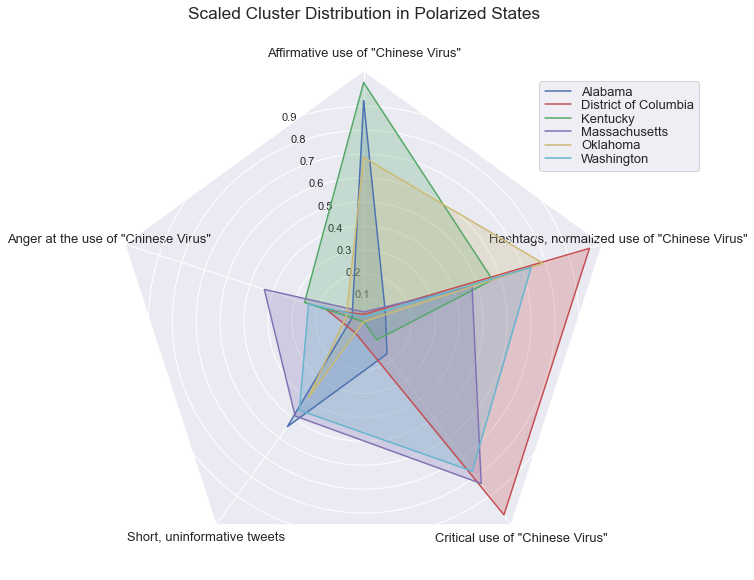
What can this tell us about Tuesday’s election? Trump’s rhetoric seems to polarize along party lines. The states where criticism is the fiercest were going for Biden regardless, and the states that support it most were already acquired to his cause. Cluster distribution in swing states, however, can shed light on the public reaction he faced in the places that matter most for the election.
North Carolina, for instance, offers a muted response: the state scores high on short, uninformative tweets, as well as tweets made up mostly of hashtags. Clusters of support and opposition to Trump’s rhetoric are both underrepresented in the state. Florida, a must-win state for Trump, has the highest level of affirmative “Chinese Virus” content among swing states—although nowhere near to the scores we saw in Kentucky and Alabama. On the other hand, Pennsylvania, Wisconsin, and Michigan all present high scores in the anti-“Chinese Virus” cluster.
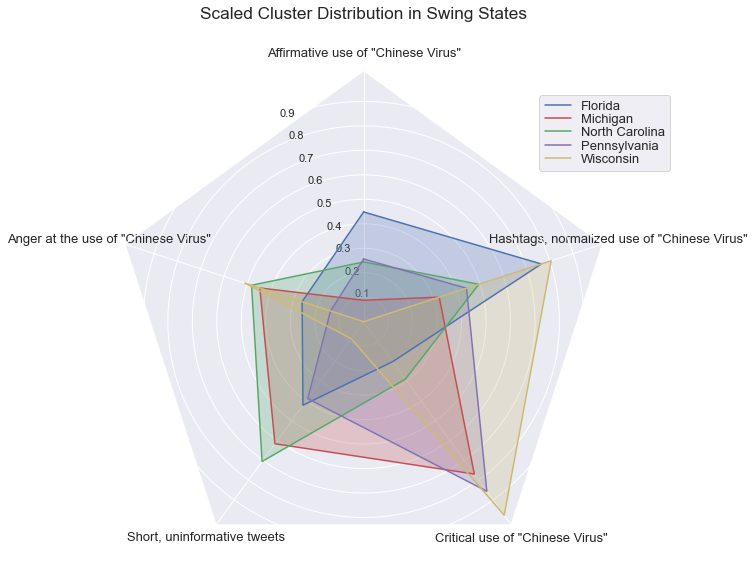
We construct an ‘Affirmation Score’ by scaling the normalized difference between the levels of the ‘Affirmative use of “Chinese Virus”’ and ‘Critical use of “Chinese Virus”’ clusters. States with scores closest to 1 are the states where affirmative use of “Chinese Virus” was the highest relative to critical use. Only states with over 100 Tweets are included in the visualization. While we don’t expect this map to look exactly like Tuesday’s electoral map, it does show that Trump’s path to victory is a complicated one given the opposition to his rhetoric we observe in several key areas (Pennsylvania, Michigan, Wisconsin, Arizona).
Pundits have long argued that Trump’s electoral strength lay in his unhinged and provocative speaking style. However, for many voters COVID-19 might have highlighted his limitations as a leader in a time of crisis—both in his management and communication. While the “Chinese Virus” term has clearly stuck within far-right groups, it does not seem to resonate with social media users in crucial swing states. If Trump does end up losing on Tuesday, his divisive and inflammatory rhetoric may very well have contributed to his unmaking.
Appendix
The code that we use for this project can be found on GitHub. The following sections walk through the data and methods we use. If you notice any bugs, or are interested in conducting other analyses on this data, please do reach out!
Data
Our analysis is conducted on a subset of the initial Twitter data accounting for all English-language tweets sent in the United States over the 4-month period (January 1st, 2020 to April 30st, 2020). We also choose to disregard “replies”, focusing instead on original posts.
We conduct two stages of cleaning on the tweet text: one preliminary step to prepare the content for BERT representation, and one more extensive step to retain only important keywords for topic classification. The initial cleaning transforms all entries to lower-case and removes website links using Regular Expressions (RegEx). We also replace some standard abbreviations (special characters such as & or contractions such as ‘rn’ for ‘right now’) by textual expressions. This way, the text retains its entire semantic meaning, and can be properly encoded using the BERT transformer. However, much of the semantic language is useless for topic assignment, and the second step of cleaning removes all non-alphanumeric characters, as well as English stop-words and one-letter words. The remaining tokens are stemmed using the nltk
Snowball Stemmer.
Subsetting to the topic of interest (“Chinese Virus”) is done using string matching on a list of keywords. Here, we use the terms “Chinese Virus”, “China Virus”, “Chinese Coronavirus”, and “China Coronavirus”, as well as hashtag versions of the terms. Over the 4-month period, we identify 14,984 topical tweets.
Methods
After subsetting on the tweets of interest, we embed them into a vector space using BERT. BERT or Bidirectional Encoder Representations from Transformers uses a transformer architecture to embed sequences of arbitrary length word by word. We use the BERT implementation fine-tuned on the task of encoding the semantic meaning of a sentence found in Reimers, et al.’s Sentence-BERT (or S-BERT), and implemented in the opensource framework sentence-transformers
. This Python package creates sentence embeddings using the average of the individual word embeddings in the sentence and trains the base BERT model on a corpus of labeled sentence interpretation data.. Specifically, it is trained as a “siamese network” encoding two sentences simultaneously to identify how similar the sentences are. We simply use one side of this network to embed each sentence individually based on its semantic meaning.
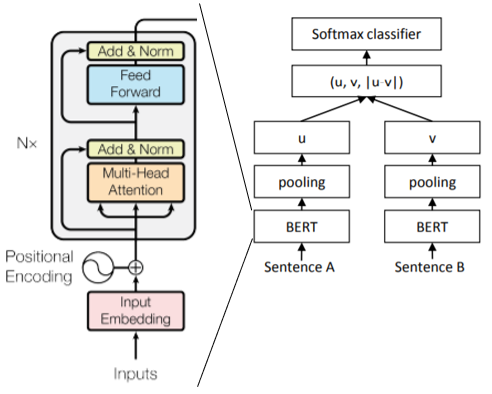
Model architecture of Sentence BERT Embedding model. Source: Reimers and Gurevych (2019), Vaswani et al. (2017)
Since S-BERT tweet embeddings are high-dimensional (1024 dimensions in the case of the pretrained model we use), we use Principal Component Analysis (PCA) for dimensionality reduction. We retain the first 100 dimensions of the PCA, which account for over 80% of the data variance.
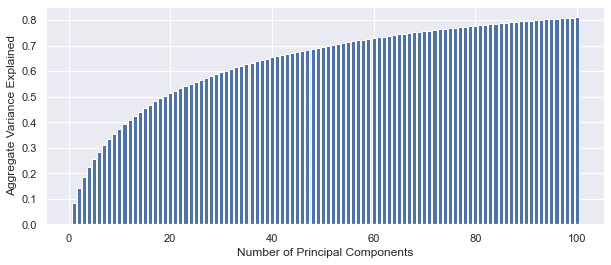
BERT sentence embeddings are clustered using the k-means algorithm. k-means initializes a set number of centroids randomly and uses Lloyd’s Algorithm to update their locations so that the distance from every point to its nearest centroid is minimized. We rely on the scikit-learn
implementation of both of these clustering methods. Since k-means is wholly dependent on the value of k, we test for different values and manually select the most relevant clustering. An alternative is to use Gaussian Mixture Models (GMM), which automatically tests different values of k and allows for more general cluster shapes. Overall, however, we did not notice a significant improvement in the quality of the results when using GMM.
The final step of our analysis is to generate a summary of every cluster based on its content. We assume each cluster’s centroid can be interpreted as that cluster’s “typical” tweet: in the absence of a BERT decoding mechanism—which would provide text associated to given coordinates—we use the 5 nearest tweets to a cluster’s centroid are an approximation of that cluster’s semantic meaning.